AI, Data 5 min read
Tabular LLMs have arrived — delivering breakthroughs for risk teams
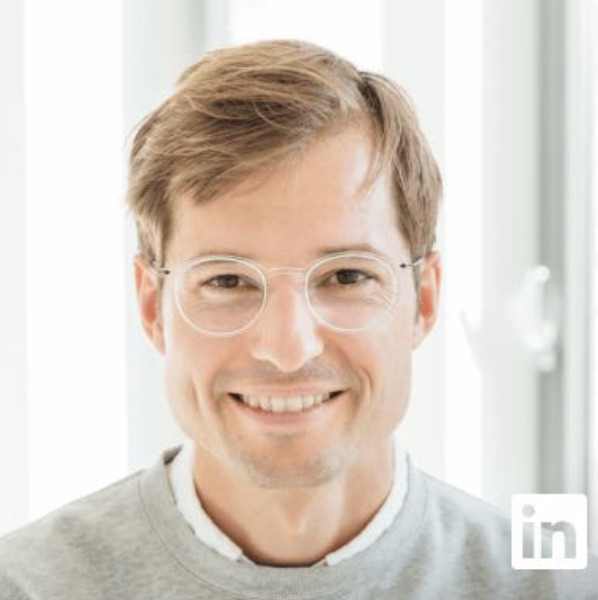
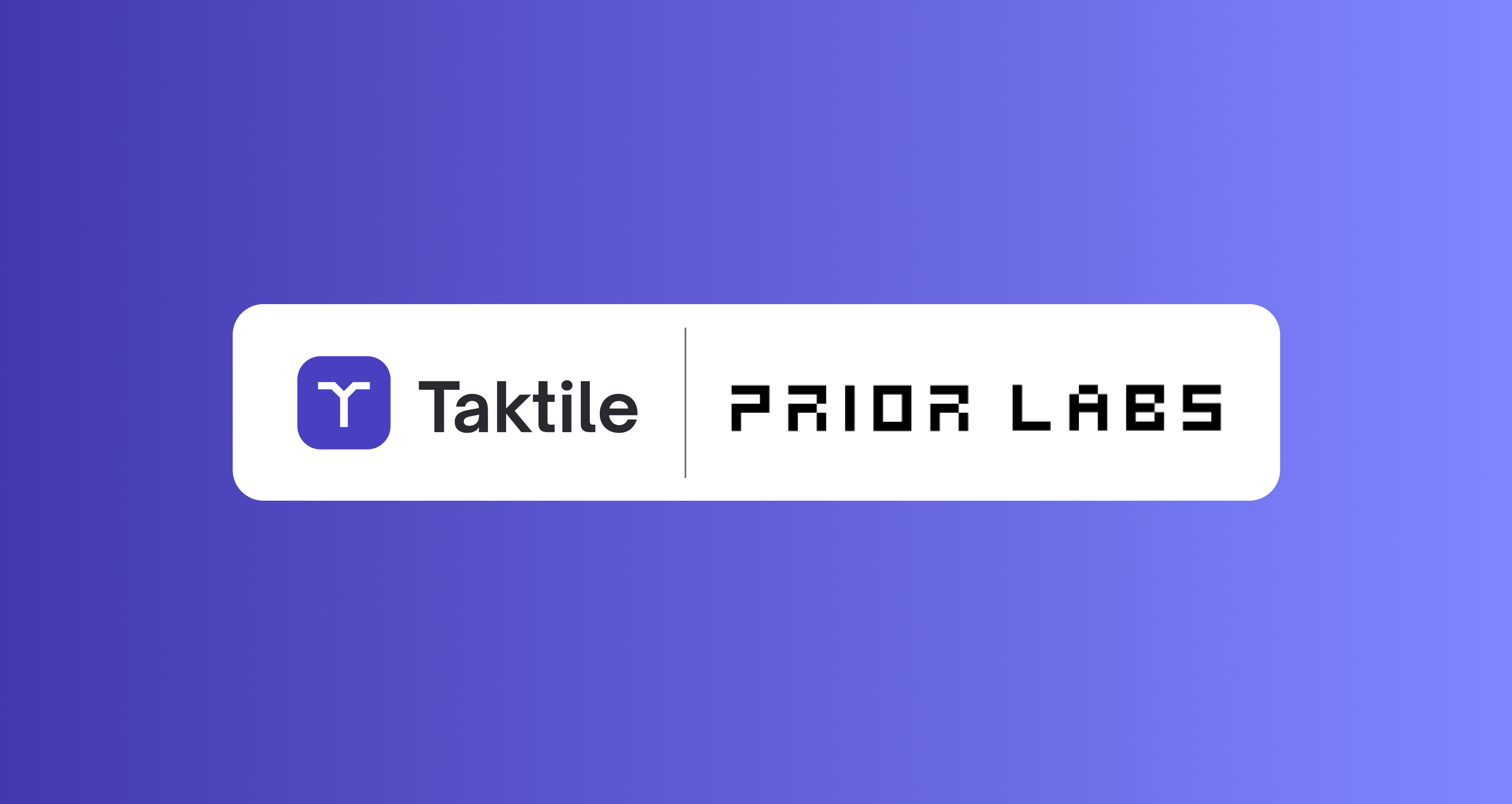
The “ChatGPT moment” of tabular data
How TabPFN works
The value of TabPFN for risk practitioners
Real-world applications of TabPFN in risk use cases
Progress in deep learning has driven breakthroughs across almost every industry — from mobility (Waymo) and biology (AlphaFold) to music (Suno.ai). However, despite the success of deep learning in highly complex tasks such as self-driving cars, risk applications have been conspicuously absent in the revolution. But this is about to change.
A key reason risk teams have yet to integrate deep neural networks into their toolkits is their historical struggle with tabular data. Banks, fintechs, and insurance companies are flush with tabular data and use it daily to prevent fraud, detect money laundering, and determine credit risk. And when it comes to building highly accurate prediction models for those use cases, the gradient-boosted trees family of models has reigned supreme.
The “ChatGPT moment” of tabular data
In a launch that industry peers have coined the “ChatGPT moment” of tabular data, Prior Labs released a breakthrough model: TabPFN. This model broadly outperforms existing methods on tabular datasets across a wide range of tabular tasks— a finding so significant that it was recently published in Nature.
Given the wide range of high-value applications across financial services, we are excited about partnering with Prior Labs to provide teams across risk, fraud, and compliance access to TabPFN.
Key takeaways
- Prior Labs has released a breakthrough model, TabPFN, that broadly outperforms existing methods on tabular datasets across a range of tabular tasks.
- The model is the new state-of-the-art in small-data regimes, and teams don’t need complex retraining and MLOps infrastructure.
- We have teamed up with Prior Labs to make TabPFN accessible on the Taktile platform for applications in risk management and decision optimization.
- The model is already proving valuable across multiple risk applications, such as fraud checks and credit risk anomaly detection.
How TabPFN works
As opposed to previous attempts at making deep neural nets useful in tabular settings, the model is pre-trained. Similar to how modern LLMs are pre-trained on a large corpus of text data, including most of the public internet, TabPFN has been pre-trained on a wide range of synthetic datasets – a key innovation of the model.
When you make a prediction with TabPFN, you give it a number of labeled rows of your data in addition to unlabeled rows. It then completes the unlabeled rows with predictions, similar to how an LLM uses the examples in a prompt to help you with a query.
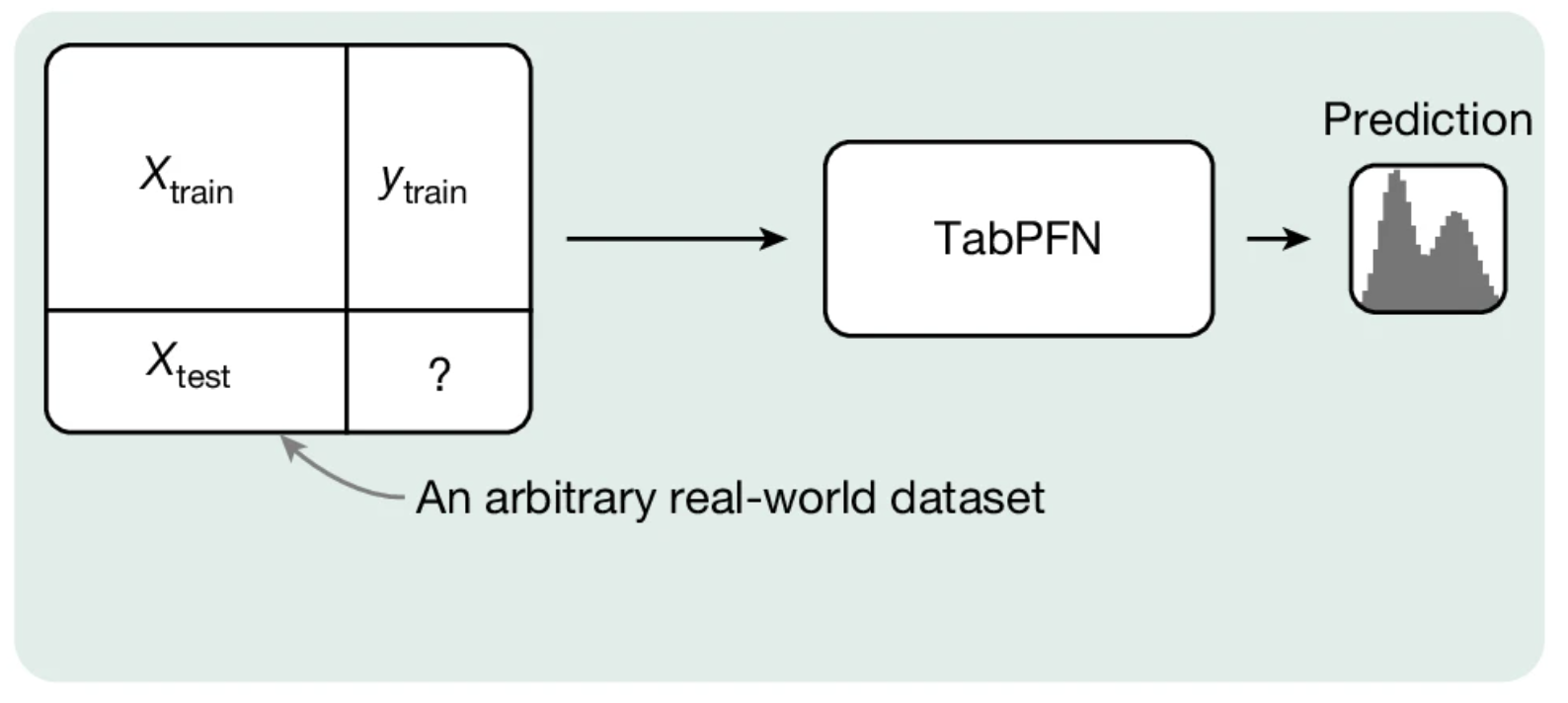
When you first hear this, it may sound unbelievable that there is no model training in the traditional sense of the word. The parameters (weights) of the model stay fixed throughout. Instead, the model relies on the flexibility and power of the transformer architecture to learn the patterns in your data while it runs the prediction. The fact that this works so well was also surprising in the early days of language modeling.
The value of TabPFN for risk practitioners
Due to the model not being trained for every new use case and heavily leaning on its priors from pretraining, there are some key advantages for practitioners in financial services:
- Domain experts can now create competitive model predictions without needing years of data science experience — this means smaller, faster, and more empowered teams.
- The model does well in small-data regimes — this is particularly exciting for fraud, credit, and anti-money laundering applications since many companies have relatively few true positives.
- Teams don’t need complex retraining and MLOps infrastructure — no retraining means you can minimize the overhead from model validation, testing, and signoff.
TabPFN also comes with valuable additional features that are often a source of friction in machine learning:
- The model naturally returns distributions for its results, which you can use in downstream decisioning.
- The model natively handles missing values and outliers, so you don’t need to think about imputation and other strategies for wresting data into the format your model expects.
- The model can return explanations for its predictions (using so-called Shapley Values), mitigating concerns around black box AI.
“We are incredibly excited about TabPFN’s ability to transform how teams work with deep learning to build and optimize financial products. The use cases we’re seeing with Taktile are really just the beginning of what’s possible.”
Real-world applications of TabPFN in risk use cases
1. Fraud checks
Given that the model performs well in small-data regimes, we think the most exciting immediate application is fraud checks. We have benchmarked the model’s performance on an anonymized, real-world account opening fraud dataset and compared it to a standard boosted trees approach. The more sparse the data gets, the larger the outperformance of the model over the classical machine learning approach. Note how the model does surprisingly well when it barely sees any training data — this is the power of pre-training in action.
Example: Fraud detection at account opening
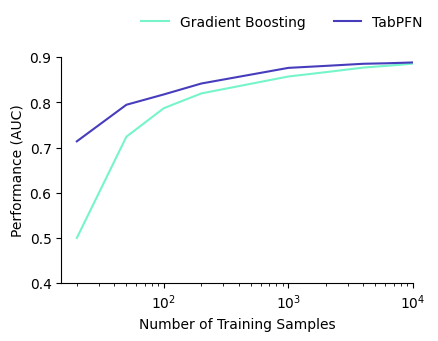
2. Credit risk anomaly detection
Another application we are passionate about is anomaly detection. The model ranks #1 in time series settings, even outperforming Amazon’s Chronos, so it can also be used to forecast approval rates and key metrics, such as the number of applications or the distribution of applicants' FICO scores. If the observed metrics drift too far from those distributions, you can raise an alert for analysts to investigate the anomaly.
Example: Anomaly detection on daily loan applications
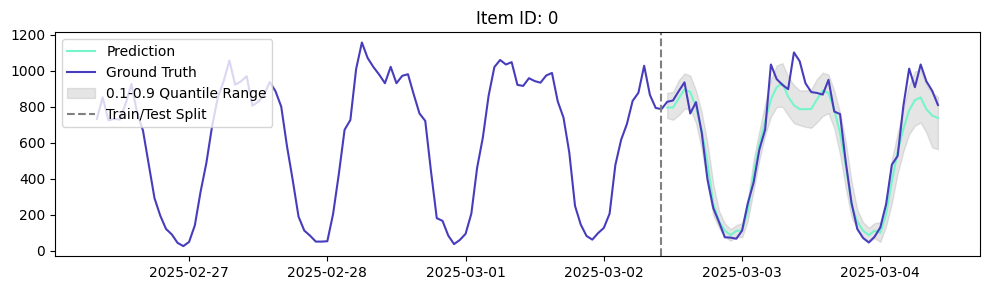
These are just two examples of why we are so excited about TabPFN – we believe there are countless more valuable use cases.
It remains important to consider limitations. Today, there is a relatively high time-to-predict (inference latency) and limitations on the supported size of data. However, we expect both limitations to soften rapidly throughout 2025.
Want to understand how TabPFN could add value to your specific use case?
Recommended additional reading
- Risk AI in action: How risk teams are building AI agents for real-world impact
- How LLMs are becoming investigative partners in fintech fraud detection
- From credit scoring to GenAI: How modern credit decisioning has evolved—and what’s next
- AI without the hype: Getting true value from LLMs without getting distracted