Data, Fraud, Lending 8 min read
Better data, better decisions: Risk assessment in the open finance era
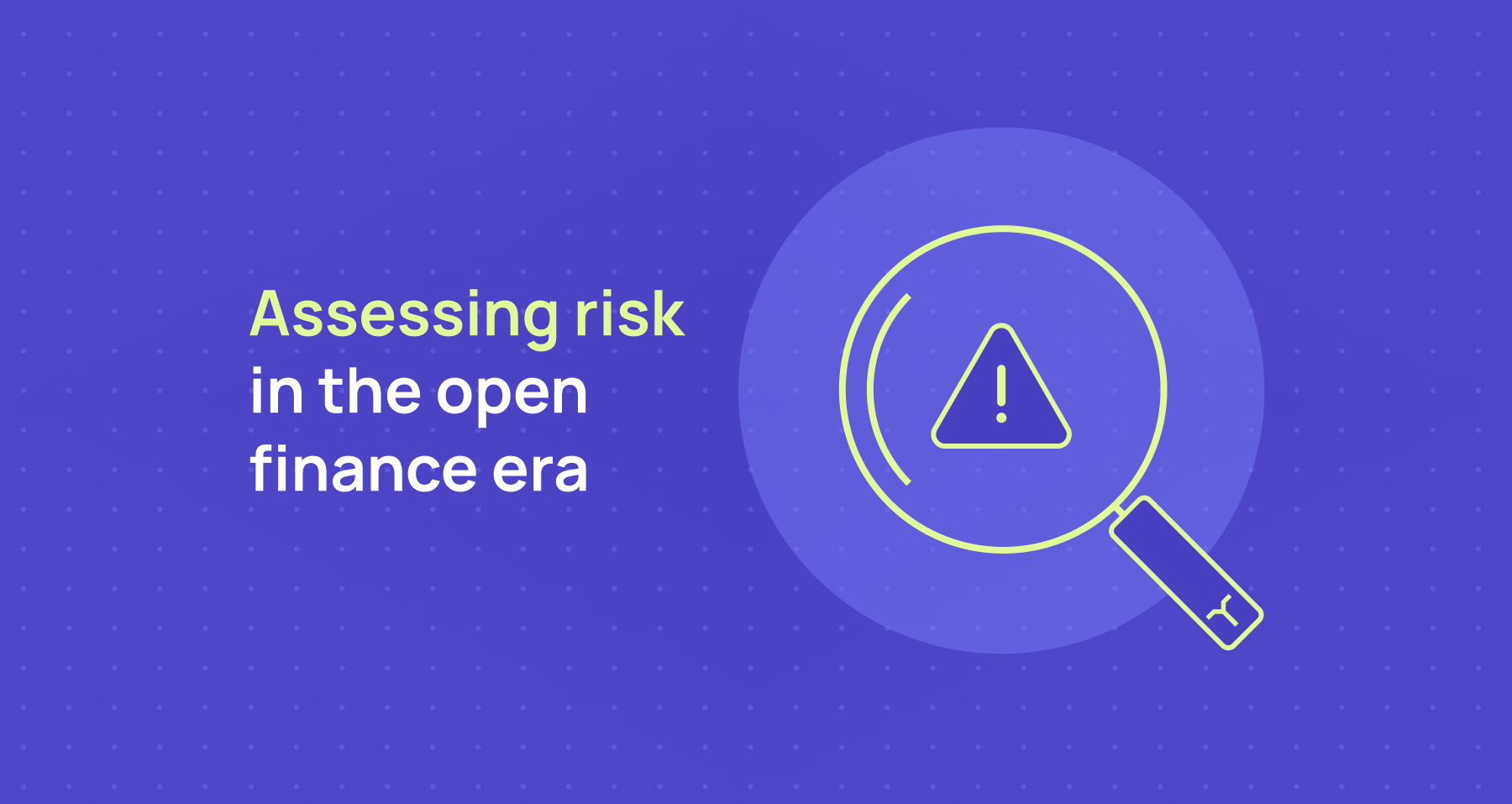
How open finance powers holistic decision-making
The benefits using open finance data for risk assessments
Three examples of open finance-powered decision-making
- Customer onboarding
- Credit underwriting
- Transaction monitoring
Risk assessment has always been at the heart of financial decision-making. However, the quality of these assessments is only as good as the data that informs them.
This article explores how open finance revolutionizes risk assessment practices, drawing on Taktile's extensive experience working with sophisticated fintech companies and banks worldwide.
It examines the new data streams available to teams today, how these enhance decision-making, and explores examples of open finance data being applied to onboarding, credit underwriting, and transaction monitoring use cases.
How open finance powers holistic decision-making
Through secure open finance API connections, product and risk teams can access a wealth of information about consenting customers’ finances—far beyond what is available in their own systems. This data powers sophisticated decision-making models, giving them a more complete understanding of customers’ finances.
Here is how this process works:
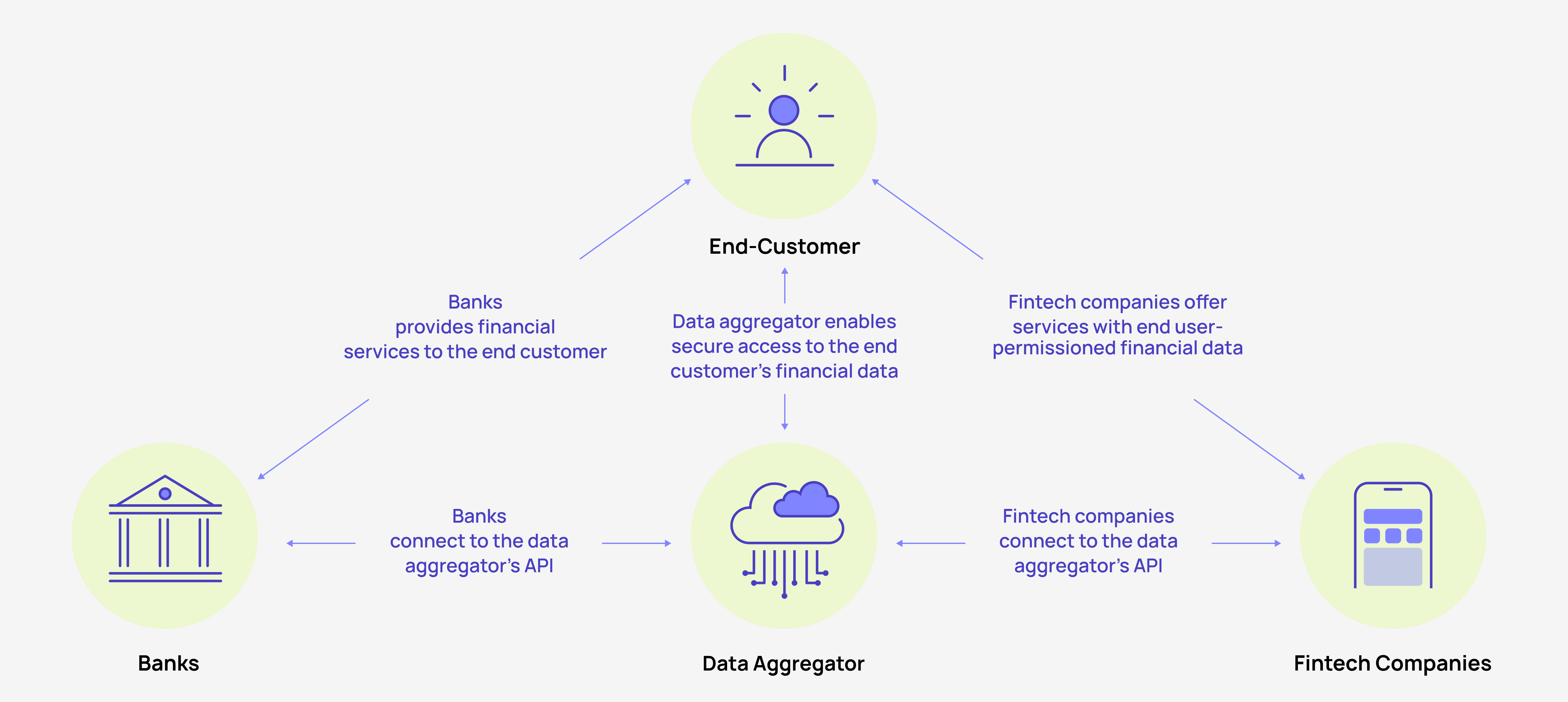
The benefits using open finance data for risk assessments
Implementing open finance data can spark a virtuous cycle of improvements for both fintech companies and banks.
Imagine a fictional mid-sized bank named FMB that has recently embraced open finance data. Here are just a few benefits that a shift towards using open finance data unlocked for FMB:
Enhanced customer insights and more accurate risk assessment
FMB began by integrating open banking data into its risk assessment process. This allowed it to gain a more comprehensive view of its customers' financial situations.
For instance, when evaluating a loan application from Sarah, a freelance graphic designer, FMB could analyze far more than just her credit score. It could also consider her consistent income from multiple clients, how she responsibly managed her business expenses, and her regular savings habits.
Tailored product offerings
This nuanced understanding of Sarah's finances enabled FMB to offer her a competitive small business loan, which it might have declined based solely on traditional credit data.
Applying this improved risk assessment across its portfolio increased its approved application rate while unlocking a significant reduction in defaults.
The success in risk assessment had a ripple effect. With more accurate risk profiles, FMB optimized its capital allocation, directing resources to promising opportunities in the freelance and gig economy markets. It also developed a suite of financial products tailored to this underserved segment, including flexible lines of credit and specialized savings accounts.
Stronger customer relationships
FMB used the wealth of open finance-powered data now available to create hyper-personalized customer experiences. For Sarah, this meant receiving proactive notifications about tax-saving opportunities based on her business expenses, and tailored advice on how to smooth out her irregular income.
This level of personalization built trust and strengthened customer relationships, significantly increasing FMB’s customer lifetime value.
Enhanced competitive advantage
As these benefits compounded, the bank found itself at the forefront of financial innovation in its market. When economic conditions shifted and many traditional lenders pulled back from small business lending, FMB was able to adapt quickly, adjusting its risk models and continuing to serve its customers effectively.
By embracing open finance, the bank transformed itself from a traditional lender into a dynamic financial partner for its customers. The result? A more agile, customer-centric, and profitable institution.
Three examples of open finance-powered decision-making
To truly demonstrate the transformative impact of open finance, we need to examine its real-world applications. This section examines three moments in the customer lifecycle where open finance is making a significant difference: customer onboarding, loan underwriting, and transaction monitoring.
1. Customer onboarding
Open finance has turned customer onboarding from a necessary tickbox process into a strategic opportunity. By leveraging open finance data, teams can create a smoother, faster, and more personalized onboarding experience—satisfying regulatory requirements while also setting the tone for the remainder of the relationship.
Streamline KYC and AML processes
Open finance has revolutionized Know Your Customer (KYC) and Anti-Money Laundering (AML) procedures. Instead of relying on manual document checks and self-reported information, fintech companies and banks can now access verified data directly from other banks and financial service providers.
For example, when a customer applies for a new account, you can, with the customer's consent, instantly retrieve their identity information, address history, and existing financial relationships from multiple sources.
This approach significantly reduces the time and effort required for identity verification. What once took days or even weeks can now be completed in minutes. Plus, by cross-referencing data from multiple sources, you can more accurately detect potential fraud or money laundering risks.
Enhance customer profiling
Open finance enables product and risk teams to build a comprehensive financial profile of new customers from day one. By accessing transaction data from existing bank accounts, investment portfolios, and credit products, teams can gain insights into a customer's financial behavior, preferences, and needs.
For instance, you might discover that a new customer regularly makes international transfers, indicating a potential need for a foreign currency account or international payment services. Or you might identify a regular savings pattern, suggesting an opportunity for investment products.
This rich data allows for immediate, personalized product recommendations, increasing the likelihood of being able to cross-sell products based on a customer’s individual requirements.
Undertake superior risk assessment
Open finance data allows institutions to conduct a preliminary risk assessment during the onboarding process itself. By analyzing a customer's transaction history, income patterns, and existing financial commitments, you can make informed decisions about credit limits, account types, and service offerings right from the get-go.
The data will be verified, up-to-date, and come directly from source institutions. Therefore, it will be far more reliable than self-reported information that may be inaccurate or outdated. This not only improves the accuracy of decision-making but also reduces the risk of errors and the need for manual corrections later in the customer lifecycle.
Personalize customer onboarding journeys
With open finance, teams can tailor the onboarding process itself based on the customer's financial profile.
For high-net-worth individuals, the process might automatically route to a premium account manager. For small business owners, it could trigger the inclusion of business banking options. This level of personalization extends to the types of information requested and the products offered, creating a more relevant and engaging onboarding experience.
Here, it becomes important to have robust but flexible onboarding infrastructure in place so that you can build, test, and optimize onboarding flows that can be easily segmented depending on the type of customer.
Continuously onboard and unlock instant account opening
Using the wealth of data available through open finance, you can implement a "continuous onboarding" model. In this approach, customers can open basic accounts with minimal information and then gradually expand or upgrade them as more data is shared and analyzed over time.
For example, a customer might open a basic savings account instantly using just their ID and consent to share their existing bank data. As you analyzes their transaction history and financial behavior over the following weeks, they might automatically become eligible for a credit card or loan product, without needing to go through a separate application process.
2. Credit underwriting
Open finance has also transformed the credit underwriting process, enabling product and risk teams to make more accurate, nuanced, and timely credit decisions.
Leveraging a wealth of real-time financial data, lenders can now assess risk more comprehensively, leading to better outcomes for them and their borrowers.
Improve your underlying risk models
The wealth of data provided by open finance allows teams to develop more sophisticated risk models. They can also use this data to power rules-based models built on next-generation risk decision platforms.
How does this work?
Machine learning algorithms can analyze vast amounts of transactional and behavioral data to identify subtle patterns and correlations that traditional underwriting might miss. Therefore, these advanced models can more accurately predict default risk, allowing lenders to fine-tune their underwriting criteria.
Unlock new methods of credit assessment
Open finance is particularly powerful in assessing creditworthiness for individuals with limited traditional credit history, such as young adults or recent immigrants. By analyzing bank transaction data, lenders can evaluate an applicant's financial responsibility even in the absence of a long credit history.
For example, a lender might consider factors like consistent rent payments, regular savings deposits, or responsible use of overdraft facilities as positive indicators, even if the applicant has never had a credit card or loan before.
Create hyper-personalized pricing and terms
In addition, open finance data enables a shift from broad risk categories to individualized risk assessment. This allows for more personalized pricing and loan terms. Instead of offering the same interest rate to everyone in a particular credit score range, lenders can now adjust rates based on a myriad of factors specific to each borrower.
For instance, a borrower with a good credit score but high utilization of existing credit lines might be offered a slightly higher rate than someone with the same score but lower utilization.
Conversely, someone with a lower credit score but consistent history of on-time bill payments and stable income might qualify for better terms than traditional underwriting would suggest.
With open finance-powered data, lenders can gain a complete view of a borrower's existing debt obligations across multiple institutions. This allows them to more accurately assess debt-to-income ratios and overall financial stress. It also helps prevent over-indebtedness by identifying borrowers who might be taking on too much debt across multiple lenders.
Make underwriting decisions in real-time
Open finance enables real-time data access, allowing for instantaneous underwriting decisions. Gone are the days of waiting weeks for loan approvals. With open banking APIs, lenders can pull and analyze up-to-date financial information at the moment of application.
Take the example of a small business owner applying for a loan. They can grant access to their business banking and accounting software data, and the lender can leverage tools like Pave.dev and Taktile to assess recent cash flows, outstanding invoices, and seasonal trends to make a well-informed decision in minutes.
Enhanced fraud detection
The rich data provided by open finance also enhances fraud detection in the underwriting process. By analyzing patterns across multiple accounts and institutions, lenders can more easily identify suspicious activities or discrepancies that might indicate fraudulent applications.
For instance, sudden large deposits just before a loan application, inconsistencies in declared income versus actual bank inflows, or unusual patterns of account openings and closures across multiple institutions could all be red flags that are more readily apparent with open finance data.
Incorporate dynamic underwriting approaches
Furthermore, using open finance data powers a shift from static to dynamic underwriting. Instead of assessing a borrower's creditworthiness at a single point in time, lenders can continuously monitor financial health and adjust terms accordingly. This is particularly valuable for products like lines of credit or long-term loans.
Imagine a borrower's income increases significantly, or they consistently maintain higher account balances. In this event, the lender might automatically increase their credit limit or offer a rate reduction. On the other hand, if financial stress indicators appear, the lender could take proactive measures to mitigate risk.
3. Transaction monitoring
When it comes to transaction monitoring, open finance data provides unprecedented visibility into customer financial activities across multiple accounts and institutions.
This enhanced perspective enables more effective fraud detection, improved anti-money laundering (AML) compliance, and better customer service.
Gain a holistic view of financial activity
In the open finance ecosystem, transaction monitoring is no longer limited to activities within a single institution. With customer consent (and within the allowed data storage timeframes), teams can now monitor transactions across multiple accounts, providing a comprehensive view of financial behavior.
This holistic perspective allows risk teams to more accurately detect anomalies, provides improved context into what is and what is not suspicious, and gives them a better understanding of money flows. This also significantly enhances AML efforts. Teams can more easily trace the origin and destination of funds, identify shell company structures, and detect patterns indicative of money laundering.
Enhance behavioral analysis and predictive monitoring
With access to a broader range of financial data, teams can conduct more sophisticated behavioral analysis, enabling predictive monitoring that flags potential issues before they escalate.
For instance, subtle changes in spending patterns or account usage across multiple institutions might signal an increased risk of default or financial distress, allowing risk teams to take proactive measures.
Additionally, the rich data provided by open finance enhances pattern recognition in transaction monitoring. Machine learning algorithms can analyze vast amounts of transactional data across multiple accounts to detect subtle patterns indicative of fraud or money laundering.
Optimize customer segmentation
Open finance data also enables a more nuanced customer segmentation for transaction monitoring purposes. Instead of applying the same monitoring rules to all customers, institutions can create more granular segments based on comprehensive financial profiles.
For example, a freelancer with irregular income patterns might have different monitoring parameters than a salaried employee with steady monthly income.
Undertake real-time monitoring and rapid response
Importantly, open finance data unlocks real-time access to transaction data, allowing for immediate detection and response to suspicious activities. This speed is crucial in preventing fraud and minimizing financial losses.
For example, suppose a customer's account suddenly shows an unusually large withdrawal. In this case, a risk team can instantly check if there is a corresponding deposit in another linked account or if it truly is an anomalous activity requiring immediate action.
Embrace the future of real-time decision-making
Open finance has ushered in a new paradigm for financial decision-making. Today, fintech companies and banks can access a wealth of real-time financial data across multiple accounts and institutions, using this to make more informed decisions throughout the customer lifecycle.
However, effectively implementing open finance requires powerful tools that can harness its full potential. This is where Taktile's next-generation decision platform comes into play. Taktile enables credit, compliance, and risk teams to effortlessly build, test, and optimize automated risk decisions regardless of their technical expertise. This includes being able to plug and play high-quality third-party data sources from Taktile's Data Marketplace of open banking aggregators, such as Plaid and Codat, to enhance decision accuracy and speed.