AI, Lending 3 min read
Money20/20 Vegas Panel Recap: ChatGPT can write, but can AI underwrite?
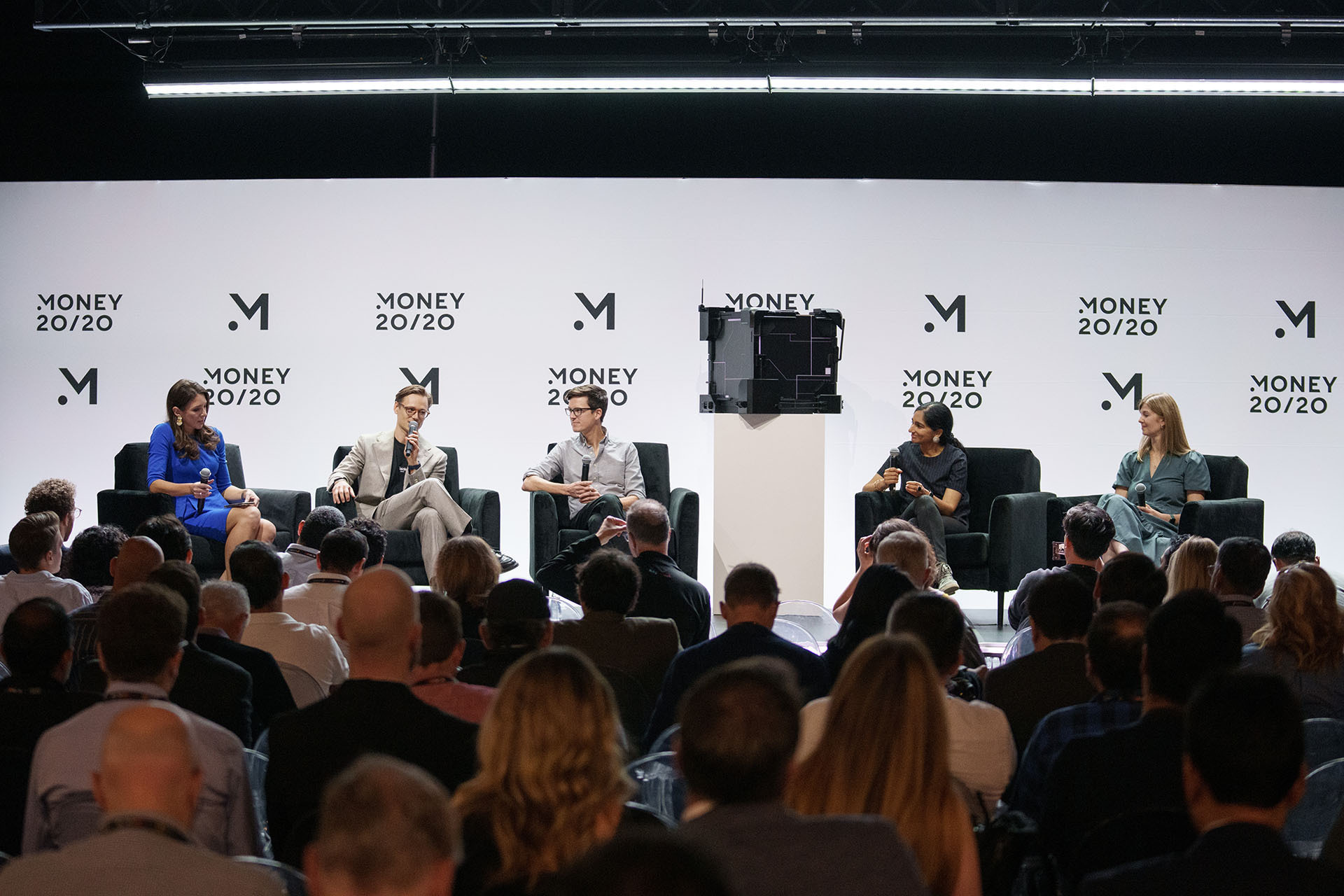
Since its release, ChatGPT has captured the world's attention, igniting discussions on how it could potentially revolutionize the financial services industry – especially credit underwriting.
Last week, I had the pleasure of discussing the challenges and possibilities of using Artificial Intelligence (AI) in credit underwriting at Money20/20 Vegas alongside three industry leaders in the space:
- Sarah Hinkfuss, a Partner at Bain Capital Ventures, a VC firm investing in ambitious growth-stage companies across fintech, application software, and climate tech.
- Matt Mollison, the Chief Data Scientist and Machine Learning Architect at Branch International, one of the world’s largest microlenders.
- Seema Amble, a partner at Andreessen Horowitz, a global VC investing in the future of b2b software and fintech.
Here are the key takeaways from our discussion:
1. Because of its inaccuracy and risk, ChatGPT will not replace the existing risk models of underwriters any time soon
ChatGPT is a product from OpenAI, one of the world’s leading AI companies. It is an interface that allows users to prompt or talk to OpenAI’s large language model (LLM) GPT-4. Because GPT-4 is trained on a vast amount of data (essentially the entirety of the public internet), it contains a lot of knowledge, and by the nature of generative AI, it is able to create net-new content.
However, generative AI, as used in ChatGPT, is only a sub-category of AI and does not represent all AI algorithms. AI and Machine Learning algorithms have been used in underwriting for years already – a long time before ChatGPT’s release.
In my opinion, ChatGPT will not be replacing the existing risk models that lenders are currently using any time soon. However, I’m very bullish on the ability of machine learning, in general, to make more accurate risk decisions.
Sarah confirmed that because LLMs like ChatGPT are trained on public data and have not seen any private default data before, making underwriting decisions using ChatGPT would be highly inaccurate and risky.
Matt emphasized that underwriting decisions also cannot be made in a black box. You need to understand the reasons behind these decisions in order to be the true owner of your decision-making logic.
Responsible Machine Learning practitioners must be able to understand the factors influencing the model's predictions. You have to be able to maintain control over the model and its training data. Models such as those used in ChatGPT learn from extensive historical data, which often contains biases – this can lead to non-compliance with regulations, depending on your operating context.
2. ChatGPT does have the power to enhance the accuracy and efficiency of risk models
Matt explained that although it is too risky to use ChatGPT for making underwriting decisions, it can be used as a tool for superhuman performance.
“At Branch, we've used ChatGPT for dataset augmentation, namely labeling, to support training our own models that we use in feature engineering, which in turn get used in our credit risk models.”
I agree that ChatGPT can be very powerful in creating new signals and variables and making risk models more accurate. I have seen a few customers already use the power of LLMs like ChatGPT to interpret and categorize banking transactions.
Previously, at Taktile, we had to hire 15 working students to help a lender label their banking transaction data. Now, we compared the results, and ChatGPT has an almost similar accuracy.
Matt explained how Chat GPT is excellent at analyzing human language. Just like in the banking transaction example, Branch can give the LLM a set of unstructured natural language data, with guidance to label different types of SMS messages, which gets a tedious task done quickly.
Seema also highlighted how valuable ChatGPT can be on the customer-facing side. It can help guide customers through the loan intake process (what things mean and where to find the data to provide), answer questions about a lending decision, and provide ongoing support on the sales and outreach side.
The real power of ChatGPT is that you can feed private data into it – even if you don’t use it specifically for underwriting decisions.
3. Outside of LLMs, AI remains incredibly powerful at predicting credit risk
Matt explained that in emerging markets, AI models have been proven to make a difference in accuracy for lenders, and Branch has been using it for years to operate at scale in its markets.
Branch operates in markets with less extensive information infrastructure than in the US, so it has to rely heavily on alternative data sources.
Instead of relying on traditional credit bureau scores to predict risk, Matt explained how they train in-house machine learning models to predict the risk associated with giving a customer a loan of a certain size.
As noted by Sarah and Seema, there are distinct differences between consumer and business lending. In developed markets, for example, it is hard to use AI for consumer underwriting decisions because of regulations, but it can be very useful in business underwriting where there is less regulation. In the US, consumer lenders are still subject to the Equal Credit Opportunity Act - which requires creditors to explain the specific reasons for taking adverse actions.
4. As the use of AI in underwriting continues to grow, so does the regulation surrounding it – lenders should prepare now for upcoming changes
AI is already being used heavily in underwriting, and we expect this to only increase in the future.
With Europe’s first AI Act already underway – many lenders, even for those not strictly employing AI in the technical sense, will need to adjust their operations to comply with both banking and AI regulation, and compliance may be required outside of the EU for anyone using AI models or outputs generated in an EU country. Therefore, lenders must start preparing now for the upcoming changes.
For a deep dive into this topic, check out our article on the future of credit underwriting under AI regulation.