Data, Fraud, Lending 4 min read
Key insights from Expert Talks with Jason Mikula: “Keeping up with the changing data landscape”
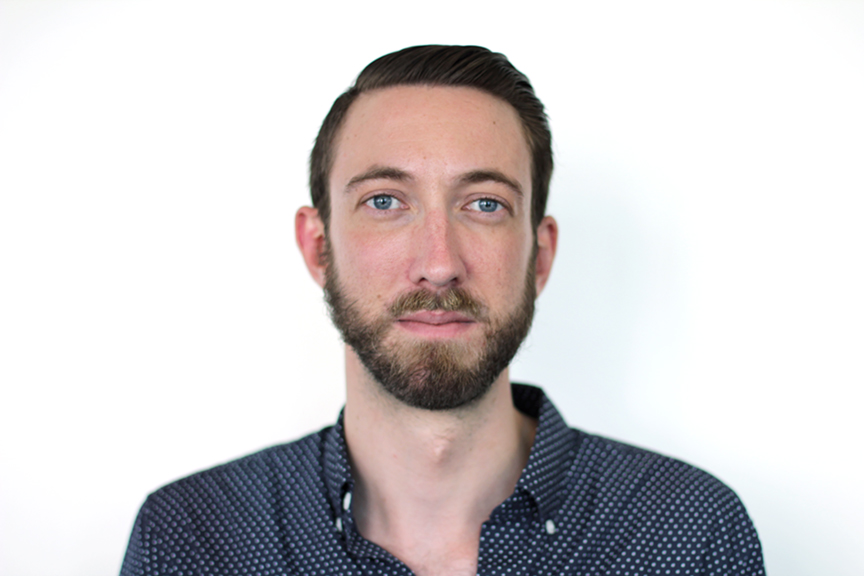
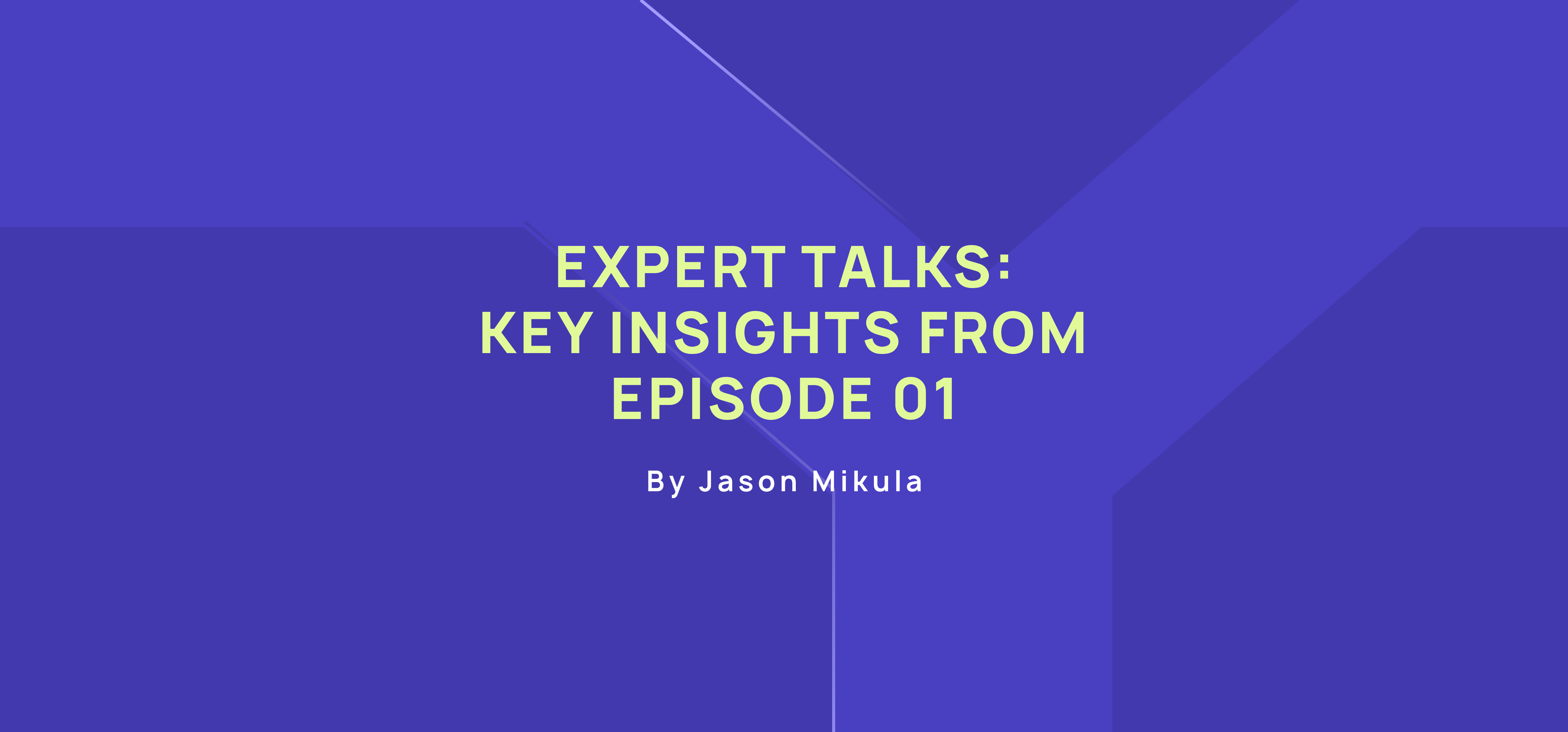
Last month, I had the opportunity to host the first installment of Taktile's “Expert Talks” series, which explored how industry leaders are keeping up with the rapidly changing data landscape.
I was joined by subject matter experts Jonathan Gurwitz, Credit Lead at Plaid, Manpreet Dhot, Chief Risk Officer at Pipe, and independent advisor and consultant Kevin Moss, who has previously held risk- and credit-related roles at SoFi and Wells Fargo.
If I had to distill the conversation into a single high-level theme, it would be that the pace of change organizations are facing today is faster than ever. The days of a “set it and forget” data strategy, regardless of the specific use case, are long over. And while our discussion wasn’t primarily focused on artificial intelligence, the opportunities to leverage AI to achieve efficiency through automation and make sense of unstructured data are readily apparent. On the flip side, AI poses risks, including around explainability and bias, and, in the hands of bad actors, is likely to elevate the threat of scams and fraud that financial organizations face.
Here are some of the key takeaways from our discussion:
1. Legacy data sources have structural limitations
Anyone who has worked in consumer lending in the United States will be extremely familiar with the major credit bureaus: Equifax, Experian, and TransUnion. The bureaus, also known as credit reporting agencies (CRAs), are rich sources of structured data on consumers’ performance on their debt obligations.
However, CRA data has limitations. The data is, by definition, backward-looking, reflecting only past consumer performance. Additionally, it is a lagging indicator; creditors usually report data monthly, so if a consumer faces financial trouble—such as job loss—this may not immediately appear in bureau records. CRA data also does not reflect a complete view of a consumer’s balance sheet, showing only what they owe, without information on income or assets, which are often essential for underwriting. Moreover, it may miss certain debts, like payday or title loans, and newer borrowing mechanisms, like buy now, pay later plans, or cash advances.
2. Transaction data greatly complements traditional credit bureau data
Consumer-permissioned bank account transaction data can serve as a complement to traditional bureau data to address some of these challenges. For instance, consumers new to credit may be “thin file” or “no file” in traditional bureau records, making it hard to score them accurately. Bank account transaction data provides an alternate window into how consumers manage their finances and a mechanism by which to evaluate their creditworthiness. In fact, transaction data can speak to an applicant’s creditworthiness in ways traditional bureau data cannot. For example, those buy now, pay later plans or app-based cash advances that typically do not appear in bureau data do appear in consumers’ bank account transaction data.
Open banking-provided data can also help lenders improve their user experience by reducing friction at key points in the conversion journey. Instead of outdated methods like using microtransactions, voided checks for bank account verification, or asking users to upload pay stubs or tax forms to verify income, consumers can securely grant permission to share data directly from their bank account or payroll provider. This approach is more seamless and accurate for meeting these requirements.
3. Clear objectives are essential for identifying and evaluating new data sources
With a seemingly never-ending list of new raw and packaged data vendors, many organizations struggle with how to identify, onboard, and evaluate new data sources. The first step should be defining what problem or challenge the data source is intended to help address and building a business case around that use case. Defining a hypothesis and approaching a new data vendor with a “test and learn” mindset can help by defining what success looks like for a given use case.
Conducting a proof of concept by evaluating a sample of a vendor’s data in a sandbox or non-production environment can help organizations to better understand if a given data source is appropriate for their use case. The proof of concept should be designed to mimic the real-life production environment to the extent possible, and proof of concept results should be compared against the business case’s assumptions and goals.
Particularly if a data source is providing processed data, such as pre-packaged features, understanding the source and reliability of the underlying data is critical to being able to provide legally required explanations should applicants be declined based on these data sources.
Technical considerations also can be a constraint in onboarding and evaluating new data sources. Understanding what kind of technical infrastructure is already in place or will be required and the product and engineering resources necessary to integrate and make use of a new data source should be mapped out in advance.
4. Overcoming integration challenges requires strategic planning
How a data source’s integration impacts a customer’s journey is a key consideration in evaluating its overall impact on conversion rate and profitability. For example, when integrating with and pulling traditional bureau data, there are various approaches about how much and what kinds of inputs to collect from a user to facilitate accessing the bureau data. The more input fields (and the more sensitive the data), the more likely users are to drop off. Other types of data integrations, like open banking, may have a more significant impact on the user’s experience and journey. Typically, in open banking integrations, users must complete a series of steps to explicitly grant access to their account and specific types of data within it. While the upside is the additional explanatory power of the data users share, there can be a negative impact on the conversion rate.
Legal and regulatory risk are also key considerations in evaluating and onboarding new data vendors. In the consumer credit space in the United States, laws like the Fair Credit Reporting Act (FCRA) and Equal Credit Opportunity Act (ECOA) and their implementing regulations govern what types of data lenders can use and provide consumers certain rights. ECOA prohibits illegal discrimination against certain protected classes, including those resulting from disparate impact. External third parties, including data vendors, must be properly overseen and managed in a process known as third-party risk management, an area that financial regulators have been paying increased attention to lately.
Information security and data privacy are also key considerations when onboarding new data sources. Depending on the source and type of data, organizations may face restrictions in their ability to retain or use that data. For instance, the recently finalized 1033 rule in the U.S., which governs open banking in the country, places restrictions on so-called “secondary use” of consumer-permission transaction data, requires data consumers to obtain users’ permission to continue accessing such data annually and may require organizations to delete users’ data if they revoke their permission.
5. Flexible infrastructure is crucial in a rapidly changing environment
Every business, financial or not, faces its own idiosyncratic needs and challenges. But there is a common challenge facing every business: change. Whether that is new and changing sources of data, new or unfamiliar customer behavior, or a changing legal and regulatory environment, organizations’ ability to quickly and effectively adapt to change in their operating environment will determine “winners” and “losers.”
Having the right technology infrastructure that gives organizations the agility to more efficiently navigate changes in their environment isn’t just a “nice to have,” but rather is a must-have in today’s new normal, constantly changing world.